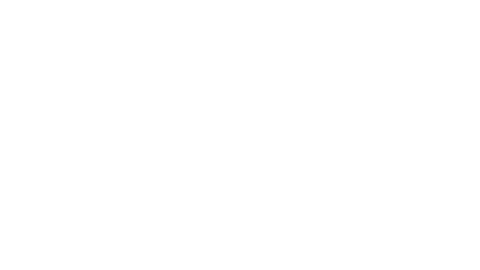
Checking your membership status...
Project Database
View Team Project Submissions for various cohorts and programs below:
52 results were found.
MAY-SUMMER 2024
TEAM
NLP stock prediction

Data Science Boot Camp
Jingheng Wang, Joseph Schmidt, Aoran Wu, Alborz Ranjbar
We design a bot trading technique based on machine learning on twitter sentiment analysis. We compare sentiment models like Vader, Naive Bayesian, and BERT to see which performs best on tweet sentiment analysis. We then use these tweets, their sentiment, and popularity of the user to assign a modified sentiment score. This modified score is one of several input features for several models that aim to calculate the best action of buy or selling a stock to maximize profit. In the end, we gain 5% advantage compared to base line model using an LSTM model.



MAY-SUMMER 2024
TEAM
Forecast Direct Normal Irradiance of Solar Energy

Data Science Boot Camp
Md Mehedi Hasan, Kamlesh Sarkar
This project aims to forecast a week ahead of Direct Normal Irradiance, which is crucial in solar energy. Nowadays, the adoption of solar energy into the power grid has increased and Direct Normal Irradiance (DNI) is particularly important in forecasting the performance of concentrating solar power (CSP) systems. Photovoltaic panels track the sun to receive more DNI. DNI accounts for a large portion of PV solar energy. So it has become essential to accurate forecasts of direct normal irradiance from solar power for the effective operation and maintenance of power systems, ensuring their ability.



MAY-SUMMER 2024
TEAM
Studying Data from the Food Environment Atlas - - GROUP 2

Data Science Boot Camp
Cyril Enyi,Mercy Amankwah,Danielle Brager,Nicole Bruce,Monalisa Dutta
Is it possible to utilize the data from the Food Environment Atlas (https://www.ers.usda.gov/data-products/food-environment-atlas/) to examine the determinants of a community's access to affordable and healthy food?
Exploring the connections between specific factors and their impact on typical food habits in communities could yield fascinating insights.



MAY-SUMMER 2024
TEAM
NSPP: News-Based Stock Price Prediction

Data Science Boot Camp
Nasimeh Heydaribeni, Mahdi Soleymani
In this project, we intend to investigate whether or not the news headlines and abstracts are good predictors of stock prices. We intend to use open large language models to extract the useful features of the news to then apply various regression methods on them.



MAY-SUMMER 2024
TEAM
Geo-locator

Data Science Boot Camp
Aashraya Jha,Dante Bonolis,Zachary Bezemek,Leonhard Hochfilzer,Francesca Balestrieri
In the popular online game Geoguessr, the player is shown a random image from Google Street View and is tasked with guessing their location on the globe as accurately as possible. In this project, we seek to solve a simplified version of this problem but using a strategy often used by professional Geoguesser players: using man-made features (for example, traffic lights) to accurately guess a city.
We use the publicly available GSV-Cities Dataset, which consists of around 500k street-view images taken in 23 different cities. We then use CNN trained on the images and features extracted from the images to make our mode. The backbone of this CNN is a pre-trained model named MobileNetV2.



MAY-SUMMER 2024
TEAM
NFL Combine Analysis

Data Science Boot Camp
Dennis Nguyen, Brett Lambert
The National Football League (NFL) is one of the largest professional sports organizations in the United States. Currently, there are 32 NFL teams and each year, each attempting to maximize performance to win the Super Bowl. Because of this, the annual NFL draft is highly anticipated as it allows teams to select players eligible to leave college football in the hopes of adding talented, young individuals on team-friendly (cheap) contracts. However, there is a great deal of uncertainty in predicting professional performance.
We modeled some of the variation in prospect success as well as draft position by using eight statistics from the NFL combine.
We additionally explored the relationship between draft position and player performance and found the relative value of various player positions at different draft positions.



MAY-SUMMER 2024
TEAM
SpotPOP

Data Science Boot Camp
Melika Shahhosseini, Ali Asghari Adib
The main objective of this project is to develop a predictive classification model that can classify the popularity of Spotify tracks based on their audio features. By analyzing a dataset containing various attributes of Spotify tracks, we aim to do extensive data exploration to identify key factors that contribute to a track's popularity and create a reliable predictive system.



MAY-SUMMER 2024
TEAM
Predicting Missed Payments from Credit Card Clients

Data Science Boot Camp
Song Gao, Juergen Kritschgau
Credit card clients miss payments on their credit card debt for a variety of reasons. Being able to predict missed payments would allow banks, credit raters, and debt collectors to forecast their own operations, target interventions or financial products, and accurately appraise the value of credit card debt. In this project, we attempt to use a client’s payment history over a 6 month and demographic information to predict whether the client will miss a credit card payment next month. We used data obtained from a Kaggle competition page to train different classification models, including logistic regressions, Bayesian models, Support Vector Classifiers, K-nearest neighbor models, and decision trees. We used cross-validation and classification accuracy to compare different classification models. Our primary finding is that no model is able to accurately predict whether or not a client will miss a payment.



MAY-SUMMER 2024
TEAM
Stock price modeling and forecasting

Data Science Boot Camp
SIU CHEUNG LAM, Suman Aich, Xiaoyu Wang, Nafis Fuad
We perform stock market analysis using data from multiple stocks (including index funds and companies). Our approach is based on both statistical modeling and LSTM neural networks. For statistical modeling we use autocorrelation plots to examine trends in data and root mean squared error (RMSE) as our key performance indicator. Using the LSTM neural network we design a regression model for forecasting and a classifier to predict whether to buy, hold or sell stocks at any given day. Finally, we explore the LSTM regression model’s ability to generalize to multiple stocks, as well as its usage for multi-day forecasting.



MAY-SUMMER 2024
TEAM
Jimmy's and Joes vs X's and O's: Predicting results in college sports analyzing talent accumulation and on-field success

Data Science Boot Camp
Reginald Bain, Tung Nguyen, Reid Harris
Recent legislation has changed the landscape of college sports, a multi-billion dollar enterprise with deep roots in American sports culture. With the recent legalization of sports betting in many states and the SCOTUS O’Bannon ruling that allows athletes to be paid through so-called “Name-Image-Likeness (NIL)” deals, evaluating talent and projecting results in college sports is an increasingly interesting problem. By considering both talent accumulation and recent on-field results, our models aim to predict relevant results for sports betting/team construction. In this iteration of the project, our targets are regular season win percentage (using a season level model that we’ll call Model 1) and individual game results (with a game by game model we’ll call Model 2) in the regular season. Our datasets come from a variety of sources including On3, ESPN, 24/7 Sports, The College Football Database, and SportsReference.com.
MAY-SUMMER 2024
TEAM
Climate Predictions Using Machine Learning Approaches

Data Science Boot Camp
Maitituerdi Aihemaiti, Abuduaini Niyazi, Rexiati Dilimulati
In contrast to modern climate models, which predict that precipitation will increase as temperatures rise, the Horn of Africa has experienced severe and recurring droughts over the past few decades. The region's agriculture-based economies have suffered greatly as a result of these droughts. Therefore, the quality of long-term weather prediction has become fundamentally important. In this project, we use multiple past climate proxy records to build a machine learning model to determine whether we can predict the future climate of the Horn of Africa.



MAY-SUMMER 2024
TEAM
Applied Neural Networks

Data Science Boot Camp
Deven Gill, Ajay Aryan, Dionel Jaime
Stock Price Prediction:
Objective: To build a neural network model that predicts stock prices based on historical data.
Dataset: Historical stock price data including trading volume, company financials, and macroeconomic indicators for a specific company (AAP was used but any company could be used).



MAY-SUMMER 2024
TEAM
QED

Data Science Boot Camp
Cisil Karaguzel, Ming Zhang, Hatice Mutlu, Adnan Cihan Cakar, Matthew Gelvin
The state-of-the-art language models have achieved human-level performance on many tasks but still face significant challenges in multi-step mathematical reasoning. Recent advancements in large language models (LLMs) have demonstrated exceptional capabilities across diverse tasks, including common-sense reasoning, question answering, and summarization. However, they struggle with tasks requiring quantitative reasoning, such as solving complex mathematical problems. Mathematics serves as a valuable testbed in machine learning for problem-solving abilities, highlighting the need for more robust models capable of multi-step reasoning. The primary goal of this project is to develop a customized LLM that can provide step-by-step solutions to math problems by fine-tuning a base LLM using a large mathematical dataset.



MAY-SUMMER 2024
TEAM
Influential Actors in Communication Networks

Data Science Boot Camp
Adam Perhala, Jungbae An
An influential actor can spread information to others in a communication network, and thus change the attitudes of others by doing so. By identifying influential actors, we can track the flow of information about a policy or product and the resulting attitudinal changes, and utilize this influence to intervene in people's attitudes or to undermine abusive interventions. In this project, we show and test a framework for detecting influential actors in the standing committee hearings in the U.S. House of Representatives–one of the communication networks of policymakers utilizing policy-relevant expertise. The influential actors identified by our framework are consistent with the relevant literature. Our detection framework can be used to optimize decision-making that leverages communication networks such as disinformation and mobilizing attention.



MAY-SUMMER 2024
TEAM
MoonBoard Grade Classification

Data Science Boot Camp
Gautam Prakriya,Adrian Batista Planas,Larsen Linov,Prabhjot Singh
A MoonBoard is a standardized rock climbing wall - fixed holds on a wall of fixed dimensions. This climbing wall comes with an app that generates routes/problems for climbers to move up. Rock climbing routes are assigned subjective grades to represent difficulty, but given that the MoonBoard is widely used there is often a consensus around grades assigned to MoonBoard problems making them somewhat objective. The goal of the project will be to build a model that identifies the grade of a given route.



MAY-SUMMER 2024
TEAM
Topic recognition on NYT articles

Data Science Boot Camp
Ravi Tripathi, Touseef Haider, Ping Wan, Schinella D'Souza, Alessandro Malusà, Craig Franze
The project proposes to study metadata of New York Times article to detect most relevant topics and build a recommendation system based on topic similarity.
We plan to do the following:
1) Apply methods like Latent Dirichlet Allocation (LDA) and Bidirectional Encoder Representations from Transformers (BERT) to identify the most relevant topics from a corpus of about 42,000 article published over the last year
2) Draw insightful visuals to highlight topic and word distribution as well as popular trends
3) Use Neural Networks to assign significant labels to topics
4) Create a recommender system based on topic similarity



MAY-SUMMER 2024
TEAM
BirdCLEF

Data Science Boot Camp
Junichi Koganemaru, Robert Jeffs, Ashwin Tarikere Ashok Kumar Nag, Salil Singh
This project addresses the BirdCLEF 2024 research code competition hosted on Kaggle by the Cornell Lab of Ornithology. Participants are provided with a dataset containing labeled audio clips of bird calls recorded at various locations in the world. The competition seeks reliable machine learning models for automatic detection and classification of bird species from soundscapes recorded in the Western Ghats of India, a Global Biodiversity Hotspot. The broader goal of this endeavor is to leverage Passive Acoustic Monitoring (PAM) and machine learning techniques to enable conservationists to study bird biodiversity at much greater scales than is possible with observer-based surveys.
MAY-SUMMER 2024
TEAM
Temporal Graphs for Music recommendation systems

Data Science Boot Camp
Abhinav Chand,Tristan Freiberg,Astrid Olave Herrera
Music streaming companies seek to increase enhance the user experience by offering personalized music recommendations. Moreover, users value personalization as a top feature on a streaming service. Music preferences can be represented as a dynamic graph of users interacting with music genres over time. Our goal is to predict the music preference of a user using classical graph algorithms, statistical inference and Temporal Graph Neural Networks. We will work with the Temporal Graph Benchmark for our study and if possible we will apply our models to other real world networks.



MAY-SUMMER 2024
TEAM
Cancer Survivability

Data Science Boot Camp
Dilruba Sofia, Funmilola Mary Taiwo, Enayon Sunday Taiwo, Samuel Ogunfuye, Karla Paulette Flores Silva, Ray Lee
Unfortunately, each of us has a 1/4 chance of getting cancer. Although with advances in treatment technologies, the survival rate of cancer patients has increased, cancer still kills many people. Breast cancer is the second most diagnosed cancer and most fatal in women. The goal of this project is to develop models that can accurately classify breast cancer patient outcomes as either "alive" or "dead", based on demographic data and clinical data at the time of diagnosis.
Data: The Cancer Genome Atlas Breast Cancer (TCGA-BRCA) project through the National Cancer Institute - GDC Data Portal.
Method: We extract patient clinical information of the patients and engineer the features as necessary. Then we apply a few classification algorithms such as random forest, AdaBoost, SVC, logistic regression, K-nearest neighbor, and MLP while keeping the decision tree algorithm as our base model to predict patients' vital status.



MAY-SUMMER 2024
TEAM
Company Discourse: How are people talking about my company online?

Data Science Boot Camp
Hannah Lloyd, Vinicius Ambrosi, Gilyoung Cheong, Dohoon Kim
In the age of digital communication, a wealth of information exists in the discourse surrounding companies and their products on social media platforms and online forums. This project utilizes natural language processing (NLP) and machine learning (ML) techniques to construct predictive models capable of assessing and rating comments provided by consumers. By employing these advanced analytical methods, we aim to enhance the correctness and effectiveness of sentiment analysis in understanding and forecasting consumer behavior. This approach is computationally efficient, while maintaining contextual integrity in the data and leveraging complex analytical techniques to gauge audience sentiment through online discourse.
MAY-SUMMER 2024
TEAM
AI-powered solutions for the restaurant industry

Data Science Boot Camp
Evaristo Villaseco, Davood Dar
In the US alone, restaurants waste 25bn pounds of food every year before it reaches the consumers plate and independent restaurants are a large driver of this. This is crucial for an industry that operates with very low profit margins of 3% to 6% on average. In this project we have partnered with Burnt (https://burnt.squarespace.com), whose mission is to help restaurants automate their back-of-house operational flow: recipe management, inventory forecasting, analysis and optimization of costs. In this project, we will use time series data from restaurants to forecast menu item sales based on different factors such as day of the week, weather, holidays etc., which will help to optimize ordering decisions for maximum efficiency.



MAY-SUMMER 2024
TEAM
Continuous Glucose Monitoring

Data Science Boot Camp
Daniel Visscher,Margaret Swerdloff,Noah Gillespie,S. C. Park,oladimeji olaluwoye
The idea of the project is to predict high glucose spikes from continuous glucose data, smartwatch data, food logs, and glycemic index. The dataset consists of the following:
1) Tri-axial accelerometer data (movement in subject)
2) Blood volume pulse
3) Intestinal glucose concentration
4) Electrodermal activity
5) Heart rate
6) IBI (interbeat interval)
7) Skin temperature
8) Food log
Data is public in: https://physionet.org/content/big-ideas-glycemic-wearable/1.1.2/#files-panel



MAY-SUMMER 2024
TEAM
If You’re Single, You’re Probably a Democrat... (and other insights into US demographics and voting inclination)

Data Science Boot Camp
Fernando Liu Lopez,Arvind Suresh
Voting behaviors depend, to a significant degree, on news and events leading up to the election; these are often unpredictable and introduce variance that undermines the accuracy of election forecasts. Yet, it is common knowledge that certain demographic characteristics are strong predictors of voting tendencies (e.g., rural areas tend to vote Republican). In this project, we employ machine-learning methods to measure the predictive power of demographic characteristics (race, gender, education, socio-economic status, marital status) in determining voting outcomes, focusing in particular on the US popular presidential vote.



MAY-SUMMER 2024
TEAM
Chirp Checker

Data Science Boot Camp
Andrew Merwin, Caleb Fong, B Mede, Yang Yang, Robert Cass, Calvin Yost-Wolff
The nocturnal soundscapes of late summer and autumn are replete with the familiar chirps, trills, and buzzes of singing insects. But these cryptic performers often remain anonymous and underappreciated.
The goal of this project was to build machine learning models to identify the presence of insects in sound files and to coarsely categorize the sounds as crickets, katydids, or cicadas.
Both Support Vector Classifiers and Convolutional Neural Networks were able to identify insects songs to the broad categories of cricket, katydid, and cicada with 90% accuracy or higher.
In the future, similar, more sophisticated models could be applied to filtering large volumes of passively recorded audio from ecological studies of insects and could power apps that identify insect songs to the species level.



MAY-SUMMER 2024
TEAM
Educational outcomes for children as a function of healthcare access

Data Science Boot Camp
Nicholas Castillo, Glenn Young, Anthony Kling, Ayomikun Adeniran, Edward Varvak, samara chamoun
According to the CDC, around 5.8% of grade school students missed at least 15 days of school in 2022 due to health-related reasons. Chronic absenteeism results in students missing milestones in reading and math, and consequently falling behind their peers, possibly putting educational success out of reach. The goal of this project is to determine if there is a relationship between ease of access to healthcare in children and educational outcomes.
Using data from the National Survey of Children's Health, we identified 88 relevant features, later refined to 10 key predictors through selection methods. We looked at various models and ultimately chose logistic regression for its interpretability and performance.



MAY-SUMMER 2024
TEAM
Headlines and Market Trends: A Sentiment Analysis Approach to Stock Prediction

Data Science Boot Camp
Jem Guhit, Sarasi Jayasekara, Nawaz Sultani, Timothy Alland, Ogonnaya Romanus, Kenneth Anderson
Financial markets are often affected by sentiment conveyed in news headlines. As major news events can drive significant fluctuations in stock prices, understanding these sentiment trends can provide important insights into market movements. This project aims to answer the question whether the sentiments extracted from financial news headlines can predict stock movements.
We use 5 years worth of data extracted from Yahoo Finance and Stock News API, obtain sentiment scores using FinVader, and use Models: Logistic Regression, Gradient Boosted Trees, XGBoost, and LSTM, to predict whether the next day's stock prices would rise or fall. We use a simulated stock portfolio to evaluate the effectiveness of the models.



MAY-SUMMER 2024
TEAM
Climate-Based Forecasting of Dengue Epidemic Months: A Case Study of Bangladesh

Data Science Boot Camp
Haridas Kumar Das, Abdullah Al Helal
Dengue outbreaks have become a global concern, affecting many regions such as the Americas, Africa, the Middle East, Asia, and the Pacific Islands. Over the past two decades, there has been a notable rise in dengue cases worldwide, with significant impacts observed in countries like Brazil and Bangladesh. Moreover, in the United States, local dengue transmission has been reported in a few states, including Florida, Hawaii, Texas, Arizona, and California. Numerous studies have demonstrated the correlation between climate factors—such as temperature and rainfall—and dengue, Zika, chikungunya, and yellow fever transmission. Specifically, elevated temperatures have been linked to an increased dengue infection risk, while extreme rainfall events have been shown to decrease this risk. In this project, we develop machine learning algorithms to analyze climate and epidemiological data in order to forecast dengue epidemic months, focusing on the analysis of Bangladesh.



MAY-SUMMER 2024
TEAM
Imputing missing data from stock time series

Data Science Boot Camp
Khanh Nguyen, Yizhen Zhao, Evgeniya Lagoda, Himanshu Raj, Carlos Owusu-Ansah, Sergei Neznanov
Missing data is a typical problem in science research. For example, in clinical trials, wearable sensors might lose signal due to battery. Errors in measuring instruments often leading to a gap in time series. Naively dropping missing data can remove important information. In this project, we investigate imputation of missing financial times series data in particular stock time series. We analyze a toy problem where we delete a few data points by hand and attempt to impute it through various methods. The goal is to see which methods and what market indicators work best for such a dataset. The completeness of stock data allows us to test how well a model predicts missing data. Analyzing imputation for such time series could therefore yield insight on correlations in international market and the relevant models and market predictors to use for the more practical problem of making forecast in price movements.



MAY-SUMMER 2024
TEAM
Doggy Doggy What Now?: Using Machine Learning to Predict Animal Shelter Intakes and Outcomes

Data Science Boot Camp
John Harden, Claire Merriman, Angela Kubena, Jun Lau, Robert Young
The Humane Society states that over 3 million dogs enter animal shelters around the United States each year, and around 2 million dogs are adopted each year. Shelters are understandably busy, noisy, and fast-moving places where many challenges present themselves. The ability to correctly anticipate how the coming days, weeks, and months will go would allow shelter managers to allocate resources more effectively. Our group sought to leverage machine learning tools and 100,000s of observations over the last decade to predict animal shelter intakes, outcomes, and adoptions. We developed time series models which include macro-level features and can predict the number of intakes and outcomes per day, week, and month with over 90% accuracy. Additionally, we achieved over 70% accuracy exploring how random forest can be used to get a paw up on predicting adoption rates with shelter-level features.



MAY-SUMMER 2024
TEAM
Predicting mental health treatment decisions from social media

Data Science Boot Camp
Eunbin Kim, Alejandra Dashe, Emelie Curl, Mitch Hamidi, Gabriel Khan
Using classification and Natural Language Processing (NLP) to analyze web-scraped data from Reddit, can we (1) identify who is undergoing or interested in mental health treatment, and (2) predict preference for treatment?
Data Source: A Kaggle dataset of scraped Reddit posts and a team-created dataset of scraped comments from eight BPD-relevant subreddits using 89 keywords of interest.
Method: 1st within the BPD community, can we classify treatment relevant content based on the text data.
2nd can we identify predictors that of BPD individuals' preference for specific treatment plans/outcomes (e.g., demographic information, comorbidity). Classification, predictive modeling, NLP,



MAY-SUMMER 2024
TEAM
Tornado Alleycats

Data Science Boot Camp
Eric Britt, Erlang Surya, Matthew Mohr, Nirdesh Bhandari, Maksim Kosmakov, Tejaswi Tripathi
Changes in the air currents and weather patterns due to climate change have shifted the location of the region of the United States that sees the most tornadoes (Tornado Alley). This migration has been towards more densely populated regions of the southeastern US where storm infrastructure is less prepared for this kind of extreme weather.
Ideally, this would show where the clouds of tornado risk shift across the US and if those regions are traveling from rural areas to more densely-populated suburban and urban areas



MAY-SUMMER 2024
TEAM
Seeking Thunder

Data Science Boot Camp
Michael LaCroix, Samson Johnson, bahareh baharinezhad, Joshua Pfeffer, Atharva Patil
We revisit a trading strategy proposed by Lynch et al. (2019) that aims to take advantage of the strong correlations in price action between constituent stocks of Exchange Traded Funds (ETFs). When an ETF experiences a high-volume negative-return day of trading, potentially from some event relevant to a subset of the constituent stocks, the stocks contained in the ETF show higher correlations among themselves than on an average trading day. Some stocks within the ETF may not be fundamentally impacted by the event, and if one suspects these “outsider stocks” to return to their baseline value it provides a profitable opportunity to purchase them at a discount. We implement the strategy proposed by Lynch et al. on more recent data and we recover the same observed increase in correlated price action among ETF constituent stocks. However, we do not recover the same alpha as the authors found in putting their strategy to practice.



MAY-SUMMER 2024
TEAM
Intelligent Recipe Suggestion System For Zero-Waste

Data Science Boot Camp
Deniz Genlik,Sevim Polat Genlik,Chun-hao Chen,Sanjay Kumar
The world wastes approximately 2.5 billion tons of food every year. This project aims to mitigate food waste by suggesting recipes based on the ingredients users have at home. The system prioritizes using items that are close to their best-before dates to reduce food waste and help users save money, while also taking users' preferred cuisines into account.
To achieve this, we compared different algorithms (KNN, Linear SVC, Random Forest) to develop a cuisine predictor for a given set of ingredients. We decided to use Linear SVC after cross-validation. Additionally, we calculated the correlation between different cuisines based on the frequency of ingredients used in their recipes. Using this correlation, we defined a distance function between cuisines and obtained a dendrogram, which allowed us to cluster cuisines methodologically. We integrated these components to develop our software.



MAY-SUMMER 2024
TEAM
Disease diagnosis using classification and NLP

Data Science Boot Camp
Rebecca Ceppas de Castro, Fulya Tastan, Philip Barron, Mohammad Rafiqul Islam, Nina Adhikari, Viraj Meruliya
Automatic Symptom Detection (ASD) and Automatic Diagnosis (AD) have seen several advances in recent years. Patients and medical professionals would benefit from tools that can aid in diagnosing diseases based on antecedents and presenting symptoms. The lack of quality healthcare in many parts of the world makes solving this problem a matter of utmost urgency. The aim of this project is to build a tool that can diagnose a disease based on a list of symptoms and contribute to our understanding of automatic diagnosis.
MAY-SUMMER 2024
TEAM
Voting: Demographics & Outcomes

Data Science Boot Camp
Yu Xiao, Srijan Ghosh, Giovanni Passeri, Li Meng
We take a look at how various relatively static socioeconomic factors affect voting patterns across the US by considering a county level breakdown of the 2020 US presidential election and modeling the results against chosen demographic indicators obtained from the US census database.



MAY-SUMMER 2024
TEAM
Flavor Finder

Data Science Boot Camp
Zhihan Li, Xue Xiao, Daniel Colon Amill, Andres Martinez, William Porteous, Michael Shteyn
Flavor Finder is a chat client that generates query-specific menu-item recommendations using Retrieval-Augmented Generation (RAG) to comb thousands of Google reviews. This process results in a natural language dish recommendation which is responsive to a user's unique dietary needs and preferences.



MAY-SUMMER 2024
TEAM
Predicting land cover using GEDI-L2A tree canopy data for New York State

Data Science Boot Camp
Frank Seidl, Luke Kiernan, Keavin Moore, Nicholas Barvinok, Noah Rahman
The tree canopy height and vertical structure of a given region can provide important implications for researchers and developers concerned with climatological trends over time. This can provide information to describe a link between important human factors, such as urban population and wildfire persistence, through an area’s land cover. We created a classification model that can predict land cover within New York state (e.g., urban-developed, forested, wetland, barren) by combining the GEDI-L2A tree canopy dataset and MRLC land cover dataset of the contiguous United States. We hope to use our best-fit model to predict land usage classifications for another region of interest, such as wildfire-prone California, or the dense forests of northern Europe.
MAY-SUMMER 2024
TEAM
PREDICTING HOUSE PRICES USING MACHINE LEARNING

Data Science Boot Camp
Herath Mudiyanselage Indupama Herath,Ersin SUER,Rafatu Salis,Sarasij Maitra
We predict house prices in King County, WA, USA using a combination of traditional features (such as bedrooms, bathrooms, and square footage) and non-traditional features (such as school ratings and crime statistics). King County was selected due to its high population density and ongoing influx of new residents attracted by major companies like Amazon, Google, and Microsoft. Our data sources include recent housing datasets from Redfin, along with school and crime ratings from SchoolDigger and CrimeGrade.org. We implement and compare various models against a baseline model to determine the most effective approach. Ultimately, we develop a Streamlit app that allows users to input data and receive housing price predictions based on our best-performing model.
MAY-SUMMER 2024
TEAM
Studying Data from the Food Environment Atlas

Data Science Boot Camp
Tatum Rask, Sayantan Sarkar, Omeiza Olumoye, Craig Corsi
Can we use data from the Food Environment Atlas (https://www.ers.usda.gov/data-products/food-environment-atlas/) to understand the factors that affect a community's access to affordable & healthful food? We used variables from the Food Environment Atlas to predict obesity rates and the prevalence of low food security. We also trained a classifier to predict whether a county is considered a persistent poverty county.



MAY-SUMMER 2024
TEAM
Cicada Zombies

Data Science Boot Camp
Wojciech Tralle, Tara Fambrough, Douglas Stauffer, Prayagdeep Parija, Henry Tucker
This spring, two broods of periodical cicadas will emerge in parts of the South and the Midwest singing their love songs. The co-emergence of these two broods has not happened since Thomas Jefferson was president. Like many animals, they, too, are susceptible to diseases. And one of those diseases they get is a fungal infection by a fungus called Massospora cicadina where a third of the body has been replaced with fungal tissue. This causes something called active host transmission causing erratic mating behavior spreading the disease (basically making other Cicada "Zombies").
It would be interesting if the Cicadas could be classified as infected vs. non-infected or possible predict impact this year. Also, a possible factor is the impact of climate change.
Initial Idea Discovery:
https://www.npr.org/2024/05/01/1248545162/a-bizarre-fungus-is-threatening-tw



MAY-SUMMER 2024
TEAM
Satisfaction Scouts - Efficient Concert Lineup Planning

Data Science Boot Camp
Anish Joseph, Vishal Bhatoy, Rachel Lopez, Obada Nairat, Eric Malitz, Peter Graziano
Problem: Concert organizers face difficulties in manually selecting and ranking bands for events.
Dataset: Spotify songs dataset found on Kaggle
Methods:
1)Data Preparation & Cleaning
2)Feature Selection
3)Similarity Calculation
4)Model Development
5)Deployment
Potential Impact: Automate the Event Planning process using data-driven recommendations



MAY-SUMMER 2024
TEAM
FinFeed

Data Science Boot Camp
Nazanin Komeilizadeh, Roberto Nunez, Korel Gundem, Diliya Yalikun, Aryama Singh
We created an AI-powered conversational bot designed to efficiently gather and present current news on finance, economy, and politics from YouTube news channels. Users can inquire about the latest economic news and receive comprehensive answers through a Retrieval-Augmented Generation (RAG) system. This system not only provides relevant information but also analyzes and displays the sentiment associated with each context of the query. Additionally, it asses public sentiments by analyzing YouTube comments related to the news topics.
We downloaded 221 videos as audio files spread over the course of 3 days from 10 YouTube channels including but not limited to Yahoo finance, Bloomberg television, The Economist, Financial Times, Reuters, The Wall Street Journal, Washington Post.
Core technologies used: YouTube Data API v3, Whisper OpenAi, Pinecone vector database, LLMs, RAG framework and Langchain



MAY-SUMMER 2024
TEAM
Predicting cancer outcomes using machine learning models

Data Science Boot Camp
Priti Singh,Pankaj Dholaniya,Ikenna Nometa,Gbocho Masato Terasaki
In this project, we aim to apply knowledge from the boot camp to analyze data from the Health Information National Trends Survey (HINTS) by the National Cancer Institute. HINTS gathers nationally representative data on the American public’s knowledge, attitudes, and use of cancer- and health-related information. This data monitors changes in health communication and technology to develop more effective communication strategies for diverse populations.
We will use survey data from the second cycle of HINTS 4, collected between October 2012 and January 2013. This dataset includes responses from 3,630 individuals across the US and contains 357 features. See hints.cancer.gov for more information.
Our investigation examines the relationship between cancer incidence and three key factors: Demographics, Utilization of Health Information Technology, and Medical History. We aim to identify features that best predict cancer outcomes using classification models and evaluate their performance.



MAY-SUMMER 2024
TEAM
Predicting bike share demand using weather data

Data Science Boot Camp
Benjamin Bruce, Keith Mills, Chutian Ma, Beni Pazar, Shaoyang Zhou
Many cities offer bike share programs, where individuals can use bikes from a shared pool on a short-term basis. The goal of this project is to predict bike share demand in Vancouver, BC using weather data, such as temperature and precipitation.



MAY-SUMMER 2024
TEAM
Stock price correlation within a sector

Data Science Boot Camp
Devadatta Hegde, Guanqian Wang, Nathaniel Tamminga, Jinjin Zhang, Keshav Sutrave, Kang Lu
Consider companies within a sector, such as banks like JP Morgan, Citi, and Wells Fargo. One company's quarterly report substantially affects the stocks of other companies. The goal of the project is to quantify this impact with some data science models.



MAY-SUMMER 2024
TEAM
N-dimensional Path Finder

Data Science Boot Camp
Dilan Karaguler, Yangxiao Luo
Algorithm that is able to explore the twisty-ness and "volume" of higher dimensional space but is able to do so with minimal amount of data points using a smart, ML way to detect boundaries (CNN with 0-value infill and kNN infill). This algorithm has wide application in data exploration where you have some criteria or in and out of bounds. This has potential application in nuclear fusion, control theory, and many other boundary type and optimization problems.



MAY-SUMMER 2024
TEAM
Classifying Sports Fans

Data Science Boot Camp
Mariah Warner, Jacob Kepes, Rudolph Perkins, Sujoy Upadhyay, Ryan Moruzzi, Deepisha Solanki
Based on self-described fandom alone, some groups may represent an untapped market that is currently overlooked. Can we, therefore, better predict different fan activities by considering self-reported fandom and various demographic data? Our goal is to identify and anticipate the populations engaging in these activities to develop more targeted marketing strategies.
MAY-SUMMER 2024
TEAM
pokemon battle AI

Data Science Boot Camp
Izabella Freitas, Guoqing Zhang, Tianyu Zhu, Hongyi Shen, Mary Collins
We aim to create a model that can predict the likely winners of competitive Pokémon Video Game Championships (VGC) battles, utilizing replay records from Pokémon Showdown!, a popular online battle simulator. This format is a highly competitive, ‘doubles battle’ where each player selects four out of six Pokémon to battle against their opponent, with two Pokémon active for each player at a time. By reconstructing these replay records into text files, we can extract turn-by-turn information from over 10,000+ battles and curate a database to train our model.
MAY-SUMMER 2024
TEAM
Forecasting Outcomes in Formula 1 Racing

Data Science Boot Camp
Edward Voskanian, Ryan Bausback, Ali Arslanhan
In each Formula 1 race weekend, three practice sessions precede the qualifying round, determining the starting grid for the race. This project aims to leverage machine learning techniques to identify key factors from practice sessions that significantly impact qualifying results. The objective is to accurately predict the outcomes of the qualifying round.



MAY-SUMMER 2024
TEAM
Is it Priced In? Classifying Efficient Markets

Data Science Boot Camp
Tanuj Mathur, Ram Purandhar Reddy Sudha, Anuvetha Govindarajan, Pinky Thomas, Neophytos Charalambides
In this project, we attempt to answer the question “Is the market aware of the situation which has taken place regarding a certain event and has it reacted to it?”. This is commonly referred to as “Priced In”, i.e. ``are all available information regarding a particular event or news, or potential outcome, already reflected in the current price of a financial asset, stock market index, or ticker symbol?''. Our approach primarily consisted of data scraping and cleaning, and training a Recurrent Neural Network. The results demonstrate that for events such as Earnings Reports, the market efficiently captures the effects through either anticipation before the event or re-adjustments after the event, and the model’s accuracy in predicting the overall effect of events (positive or negative) based on market & stock history highlights its robustness and practicality in applications.


